How to build the perfect data scientist
This article contains affiliate links. We may earn a small commission on items purchased through this article, but that does not affect our editorial judgement.
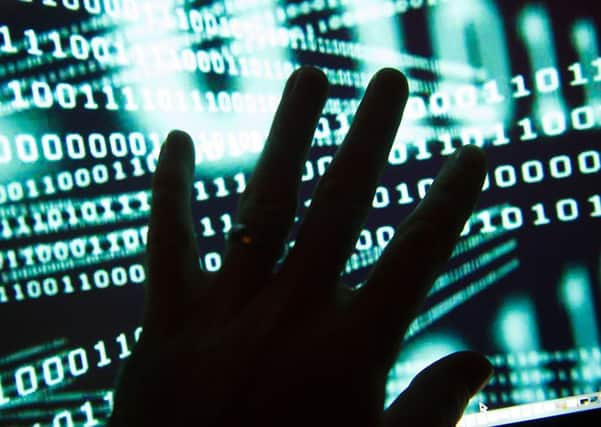
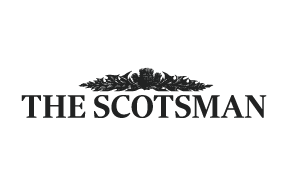
By sorting through mountains of information generated by their organisation, data scientists can identify trends and buying patterns that can improve the bottom line of the businesses that employ them. These information specialists can command impressive starting salaries and are in high demand across the UK. Here, Jonathan Forbes outlines how to become the perfect data scientist.
Contrary to popular belief, the perfect data scientist is not a robot.
Advertisement
Hide AdAdvertisement
Hide AdHe or she is not construct of artificial intelligence, machine learning and Skynet. The answer to the question of what makes a perfect data scientist is much more prosaic than that.
According to consulting firm Booz Allen Hamilton, to become the perfect data scientist one needs first to sail the seven c’s of big data analytics. These are listed as:
1. Curious and cognitive Ask questions but make sure they are the right questions.
2. Creative. Be a design thinker.
3. Courageous problem solver. Have a willingness to question the culture and be prepared to fail on occasion.
4. Cool under pressure. Remain calm and look at the ambiguity, even if the insight is not clear at first.
5. Continuous life-long learner. Stay on top of new methodology and technology.
6. Communicator. Tell others the story that the data is showing you.
7. Collaborative. Don’t work in isolation - buy into the ‘data is a team sport’ adage.
Advertisement
Hide AdAdvertisement
Hide AdAs with most things in the data science world it’s still not as simple as merely having these seven attributes. BAH adds a further three c’s: critical thinker, computational and consultative.
Stephan Kolassa published an update on Drew Conway’s famous Data Science Venn Diagram in 2014. His reinvented version moots the idea that the perfect data scientist is equal parts an expert in communication, statistics, programming and business. A very large pair of shoes to fill indeed. It would be extremely hard to try and become that person and even harder recruit them.
Data scientists need engineers and vice versa. You can’t really have one without the other. There are many famous examples of such pairings in the scientific world but perhaps the best illustration is in the world of music. Lee Dickson was Eric Clapton’s guitar tech for over 30 years but most people will not be familiar with his work although they almost certainly will be with the person handling his expertly prepared instruments. Without Dickson doing his stuff in the background Clapton would not have been as successful in delivering his product.
In the search for the perfect data scientist I believe the time has come for a change in thinking, approach and even organization. The expectations we have of this fabled data scientist are unrealistic. We must understand that data scientists and data engineers need to work closely together. And by together, I mean literally together. Not in different teams or in separate silos. I firmly believe that data analytics is a team sport.
Having said all that I do want a little bit more from my data scientist but not too much more. There are aspects of data science - methods, techniques, analytics tools and vertical applications - that are essential for a data scientist to know and have at their disposal. There are also elements that are nice to have. At Aquila Insight we make sure our scientists get training from data engineers, we help our data scientists to become as self standing as they possibly can be.
We see that there are sometimes tensions between the scientist and the engineer. Scientists often don’t know where the data is going to take them while engineers know for certain that they have to turn the data pipelines into something that can produce models and their insights. When we get a good fit of scientist and engineer it really works and we see the results.
I really believe that we will soon see the dawn of the strongly bonded data scientist and analytics engineer pair or team that move together from job to job, just like creative partnerships in the advertising world. We’ll see analytics speed up as practices become established and reusable patterns and code develop. We’ll also see scientist and engineer tools move with them. The team will bring their favoured analytics platforms and algorithms with them rather than adopting any in-house tools or process.
The perfect data scientist probably doesn’t exist outside a few lucky geniuses, but a near perfect data scientist can be built. He or she will need support and investment from their organisation in conjunction with equal or greater levels of investment in data engineering.
Advertisement
Hide AdAdvertisement
Hide AdAs a young data scientist, make sure you get that support. If it is lacking, put your career first and move to an organisation that will invest in your development for the future.
Jonathan Forbes is Chief Architect at Aqulia Insights.
DOWNLOAD THE SCOTSMAN APP ON ITUNES OR GOOGLE PLAY